Curious about the differences between AI, machine learning, and deep learning—and what they mean for education? In this talk, Jeff Crume breaks down these key concepts, explores the rise of Generative AI (Gen AI) like chatbots and deepfakes, and helps clarify how these technologies are shaping the future of teaching and learning.
Video: AI, Machine Learning, Deep Learning and Generative AI Explained (10:00)
Types of AI in Education
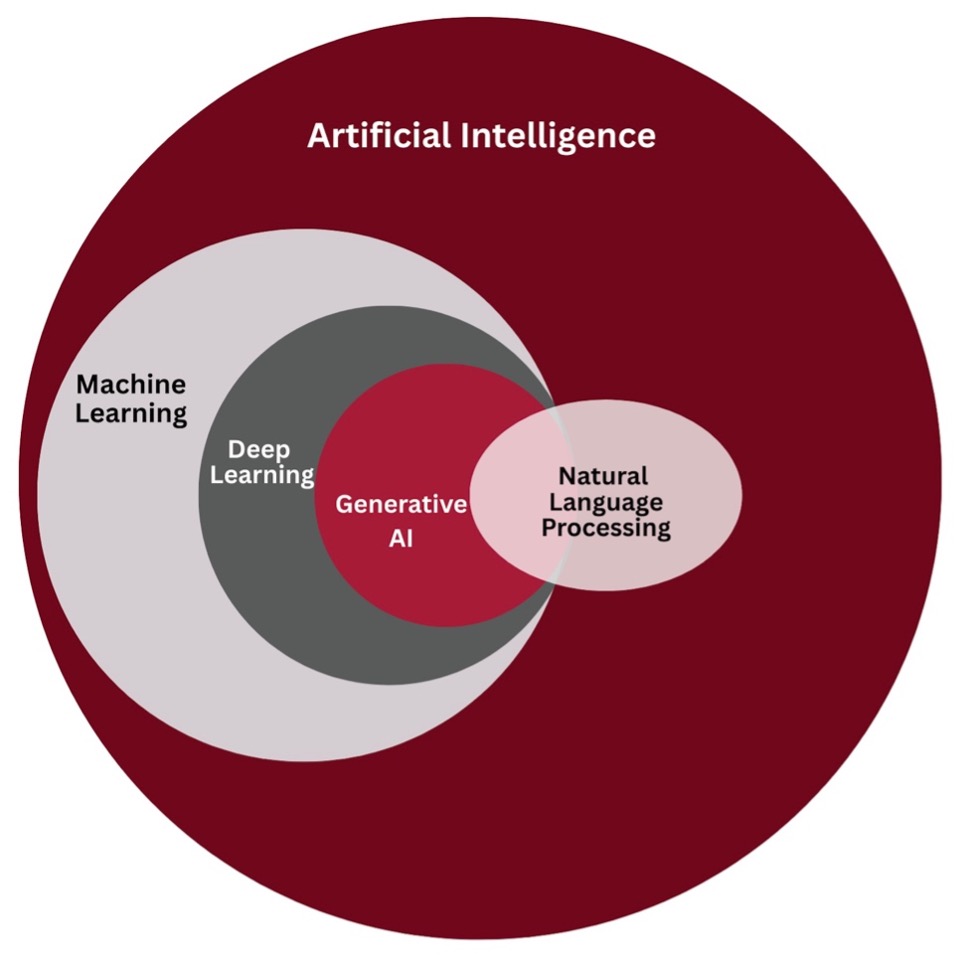
Machine Learning (ML)
systems that identify patterns in data and improve over time
- In education, this means that digital tools can adapt to individual student needs and performance, helping personalize instruction and support in real time.
- Examples include personalized learning platforms like DreamBox or Knewton which analyze student responses to tailor lessons in real-time. Also, ML can flag at-risk students by analyzing attendance, grade, and participation patterns. This information can then be used to help educators intervene earlier.
Deep Learning
uses neural networks with many layers to learn patterns and make predictions from large datasets.
- In education, deep learning allows for more sophisticated personalized learning systems that can adapt to each student’s unique learning patterns and needs.
- An example includes intelligent tutoring systems like Carnegie Learning which uses deep learning to track student progress in math and automatically adjusts the difficulty level based on performance patterns.
Generative AI
tools that create new content like text, images, or code based on prompts
- In classrooms, this opens new creative possibilities for both students and teachers, from generating story ideas and visuals to designing learning materials on demand.
- Examples include creative assignments with the use of tools like ChatGPT, DALL-E, or Canvas’s AI tool to brainstorm essays, design visuals, or prototype ideas. Teachers can generate quizzes, lesson plans, rubrics, or email drafts—all of which help save time and enhance productivity.
Natural Language Processing
technology that understands and generates human language (powers chatbots and writing assistants)
- This allows for educational applications to help students write more effectively, interact with AI tutors, or receive real-time language feedback.
- Examples include writing support tools like Grammarly or Quill that give students grammar suggestions, rephrasing help, or even tone feedback. Additionally, virtual teaching assistants like ChatGPT or other AI bots can help students answer questions 24/7, explain concepts, or summarize reading material.
AI Limitations
Video: 3 types of bias in AI | Machine Learning (2:33)
The following video provides an overview of three common types of bias that can occur in artificial intelligence systems. Understanding these biases is crucial for developing fair and accurate AI models.
Core Limitations
The video “3 types of Bias in AI | Machine Learning” highlights 3 core limitations related to bias in AI systems. These core limitations are:
- Data Bias – This is when the training data reflects societal or historical biases, it leads to skewed or unfair outcomes. For example, data bias can appear in facial recognition systems. Some facial recognition systems have been shown to perform worse on people with darker skin tones.1
- Algorithmic Bias – This is when the design of the algorithm or model introduces bias, even if the data is neutral. An example of this can be found in résumé screening algorithms. Amazon developed an AI-based recruiting tool that exhibited bias against women. The system penalized résumés containing the word “women’s” and downgraded graduates from all-women’s colleges. The algorithm was training on past hiring data that favored male candidates, leading it to learn and perpetuate gender bias.2
- Human Bias – This is when human choices in selecting data, features, or labels introduce subjective assumptions into the system. For example, an algorithm in U.S. healthcare was designed to predict which patients would benefit from extra medical care. This tool significantly underestimated the health needs of Black patients compared to white patients. The algorithm used healthcare spending as a proxy for health needs. The choice of the metric introduced human bias into the system.3
These biases can affect the fairness, accuracy, and trustworthiness of AI applications.
Top Benefits
- Efficiency and Automation – AI can automate repetitive tasks, streamline workflows, and process data at high speed.
- Data-driven Insights – AI can analyze vast amounts of data to uncover patterns, trends, and predictions that would be difficult or impossible for humans to detect. This can lead to smarter decision-making in areas like healthcare, finance, and marketing.
- Personalization – AI enables personalized experiences like tailored recommendations on streaming platforms or individualized learning paths in education. User engagement and satisfaction can be improved through personalization methods with AI.
References
1Hardesty, L. (2018, February 11). Study finds gender and skin-type bias in commercial artificial-intelligence systems. MIT News. https://news.mit.edu/2018/study-finds-gender-skin-type-bias-artificial-intelligence-systems-0212?utm_source=chatgpt.com
2Dastin, J. (2018, October 10). Insight—Amazon scraps secret AI recruiting tool that showed bias against women. Reuters. https://www.reuters.com/article/world/insight-amazon-scraps-secret-ai-recruiting-tool-that-showed-bias-against-women-idUSKCN1MK0AG/?utm_source=chatgpt.com
3Obermeyer, Z., Powers, B., Vogeli, C., & Mullainathan, S. (2019). Dissecting racial bias in an algorithm used to manage the health of populations. Science 366(6464). 447-453. DOI: 10.1126/science.aax2342